What are word vectors?
This semester I’ve been learning a lot about word vector models as a tool in analyzing large corpora of text. These models have recently become extremely popular in fields of computational linguistics and corpus analysis, as they can start predicting words that are used in similar contexts to others if they are trained correctly. For example, asking a model what the closest word to ‘army’ might be ‘military.’ However, the models can be much more powerful. Using some complicated vector math, you can ask the model what the closest between the sum of two words (usually you would be asking for the sum of their meanings) or the difference of two words. So ‘king’ + ‘woman’ or ‘king’ – ‘man’ might result in ‘queen.’ The models can even handle analogies. ‘King’ – ‘queen’ + ‘prince’ would yield ‘princess’ (semantically this query and result means ‘king is to queen as prince is to princess’).
The word vector model that I used for this research project is Word2Vec, which was created by Google. Because I was doing this project as part of an English class, our instructors created an easy script for us to run that would train a model for us. All we had to do was point the model to the correct files and run queries on the trained model. We did this all in the R programming language.
The Research Question
In order to be able to start working with word vector analysis, I needed to come up with a research question. This step of the process was probably the hardest for me, as many of the questions I wanted to answer were not actually questions that could be answered using word vectors. I ended up choosing the question of: “are older people seen as having more authority in children’s literature?” However, this question didn’t end up being the best for word vector analysis either, so I switched paths a bit. Instead I looked at how people with authority, particularly political authority, are viewed. Are they normally viewed in a positive or negative light? And, is there any difference in how women in power are viewed compared to men in power?
The Other Research
For some background information, I read two articles on the subject of my research question: “The Benevolent Bureaucrat: Political Authority in Children’s Literature and Television” by Thomas Marshall and Timothy Cook’s response, “Another Perspective on Political Authority in Children’s Literature: The Fallible Leader in L. Frank Baum and Dr. Seuss.” Both were published in The Western Political Quarterly in the early 1980s. Marshall, after looking at political figures in television and literature aimed at children, concluded that “overall, government appears in the media as a positive and benevolent force, delivering needed and sought-after services with skill and compassion…America’s child-oriented mass media produce an overwhelmingly favorable portrait of the public sector for America’s youth” (397). This research seems to suggest that characters with political authority are viewed in a primarily positive light in children’s literature. However, Cook argued that Marshall’s experiment surveyed a range of media marketed toward children, but did not take into consideration what children actually read. As Cook put it, “while a random selection of children’s books does provide a representative sample of the content of, say, a children’s section of a library, it need not give a representative sample of what children read…Some books are far more popular than others among children, who often concetrate [sic] on particular genres series, or authors” (327). He argues that in L. Frank Baum’s and Dr. Seuss’s works, political figures are often portrayed in a negative light. Because L. Frank Baum and Dr. Seuss were some of the most popular authors of children’s novels, Cook argues that we cannot disregard their nonconformity to the rest of children’s literature that Marshall studied (Cook 327).
The Corpus
Corpus preparation is one of the most important steps in word vector analysis. Because the model is trained on your specific corpus, if there’s something wrong or weird with your corpus, there’s going to be something wrong with your results. First you have to decide which texts are going to go into your corpus. Then you have to decide how you’re going to clean your data.
My corpus is comprised of 20 pieces of children’s literature from the 19th and 20th centuries. I found a Children’s Literature bookshelf on Project Gutenberg and decided to use that to get my texts in text file format. I sorted the bookshelf by popularity and took the top 20 results, as long as they followed the following rules: 1. There could be only one book from any given author in my corpus and 2. Only novels and short stories were allowed – I did not take any anthologies of works. While picking no more than one book per author goes against what Cook argued in his article, I decided that I was more interested in the general trend across children’s literature, instead of looking at specific novels or authors. However, I did err when creating my corpus, and added three books by Frances Hodgson Burnett and two stories by George MacDonald. In the end I think this was a good thing, because I had already gotten to books and stories that I had never heard of, and I would have rather had a few duplicate authors in my corpus than have novels that were not popular children’s novels. For a full list of the works included in my corpus, see Appendix A.
In terms of cleaning, I did very little by hand. The text files from Project Gutenberg come with some metadata at the beginning and end, such Title, Author, and information about the book, as well as licensing information. I simply removed all of that information from the beginning and end of each text file. Additionally, all of the works I chose had some sort of chapter naming and/or numbering system. I also removed all of the table of contents and any chapter markings. I then let our class R program run on the text files, which removed stop words (such as ‘the,’ ‘a,’ ‘of,’ etc.) and lowercased all of the words. I did not remove any character names, as I didn’t think that they would mess up my results that much (sometimes I did get character names in results, but not so much that the rest of the words that came back were invalidated). Altogether, my cleaned corpus had about 1 million words, which is the lower limit for a model trained on that corpus to give reasonable results.
The Data
In this section I will give some examples of queries that I ran on my model. For more queries that I did not talk about, see Appendix B. To start, I ran some queries on single words that related to my research question. For example, I first queried for the 20 closest words to ‘governor.’

As you can see, the results included words such as ‘flogging’ and ‘thrashing,’ but also ‘kindest.’ However, more interesting things happen when looking at the intersection of ‘governor’ and ‘power.’
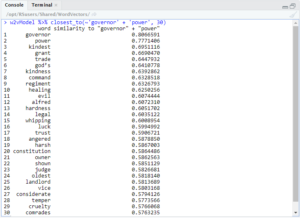
Here you can see many negative words associated with these words, such as ‘evil,’ ‘hardness,’ ‘whipping,’ ‘angered,’ ‘harsh,’ ‘temper,’ and ‘cruelty.’ Again, there are also many positive words show up, such as ‘kindest,’ ‘kindness,’ ‘healing,’ ‘trust,’ and ‘considerate.’ In fact, most queries such as this had both positive and negative words.
I decided then to try to see if there was any difference between women and men in power, and if one was viewed as more benevolent or malevolent compared to the other.The first thing I wanted to check here was ‘king’ vs ‘queen.’
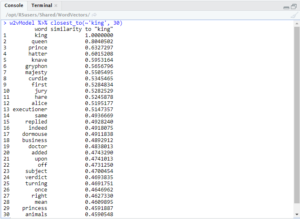
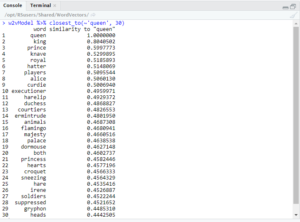
As you can see, both ‘king’ and ‘queen’ returned ‘executioner,’ but only the former returned ‘mean’ in these results. I also searched for ‘governor’ + ‘man’ and ‘governor’ + ‘woman.’
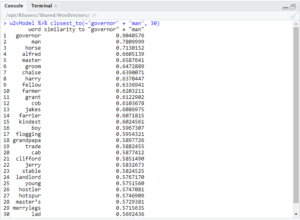

Both queries returned ‘kindest’ and ‘flogging,’ but the relative positions of those two words are different. For ‘governor’ + ‘man,’ ‘kindest’ was the 15th most similar word and ‘flogging’ the 17th. However, in ‘governor’ + ‘woman,’ ‘kindest’ was 12th most similar, and ‘flogging’ was 29th.
The Takeaways
So, what do my results mean? Well, for my first question, I would say that there is no evidence that suggests that people in power are viewed as particularly bad or good. When querying on words related to political authority, both positive and negative words are consistently returned. However, on the question of gender, I could claim that my findings suggest that women in power are seen as overall more benevolent than their male counterparts. This is because in the queries I did on men vs women, the women’s queries returned either more positive words, fewer negative words, or had positive words as more similar and negative words as less similar to the query itself. But is this enough to prove anything? Probably not. The evidence is a bit of a stretch – it’s not concrete at all. What is most likely is that my corpus is not large enough to have very accurate results. There are over 1 million words in the corpus, but this still might be too few. It looks like some more research is needed.
Works Cited
Cook, Timothy E. “Another Perspective on Political Authority in Children’s Literature: The Fallible Leader in L. Frank Baum and Dr. Seuss.” The Western Political Quarterly, vol. 36, no. 2, June 1983, pp. 326–336., doi:10.2307/448245.
Marshall, Thomas R. “The Benevolent Bureaucrat: Political Authority in Children’s Literature and Television.” The Western Political Quarterly, vol. 34, no. 3, Sept. 1981, pp. 389–398., doi:10.2307/447218.
Appendix
Appendix A – List of Works in Corpus
Title |
Author |
Year Published |
A Christmas Carol in Prose; Being a Ghost Story of Christmas | Charles Dickens | 1843 |
Alice’s Adventures in Wonderland | Lewis Carroll | 1865 |
A Little Princess | Frances Hodgson Burnett | 1905 |
Anne of Green Gables | L. M. Montgomery | 1908 |
Black Beauty | Anna Sewell | 1877 |
Heidi (Translated to English) | Johanna Spyri | 1881 |
Little Lord Fauntleroy | Frances Hodgson Burnett | 1886 |
Little Women | Louisa May Alcott | 1868 |
Peter Pan | J. M. Barrie | 1911 |
The Jungle Book | Rudyard Kipling | 1894 |
The Legend of Sleepy Hollow | Washington Irving | 1820 |
The Light Princess | George MacDonald | 1864 |
The Princess and the Goblin | George MacDonald | 1872 |
The Railway Children | Edith Nesbit | 1906 |
The Secret Garden | Frances Hodgson Burnett | 1911 |
The Story of Doctor Dolittle | Hugh Lofting | 1920 |
The Velveteen Rabbit | Margery Williams | 1922 |
The Wind in the Willows | Kenneth Grahame | 1908 |
The Wonderful Wizard of Oz | L. Frank Baum | 1900 |
Treasure Island | Robert Louis Stevenson | 1883 |
Appendix B – More Queries
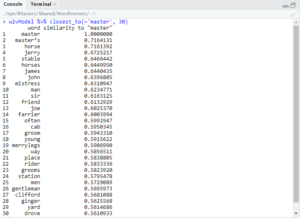
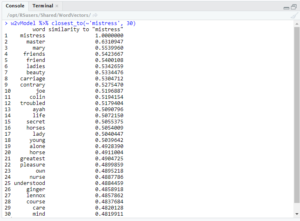
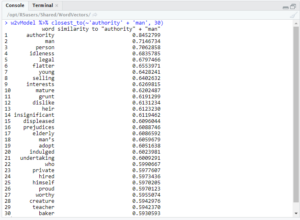
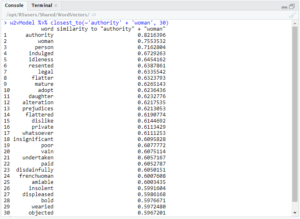
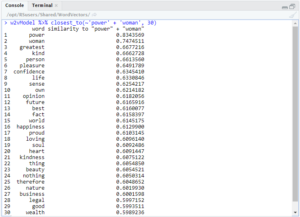
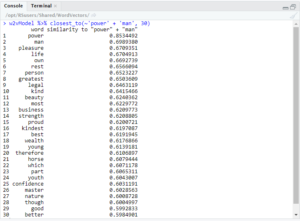
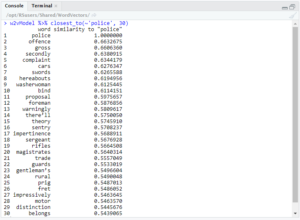